Dashboards of key drivers for the recently released IEA Sustainable Development Scenario (SDS)
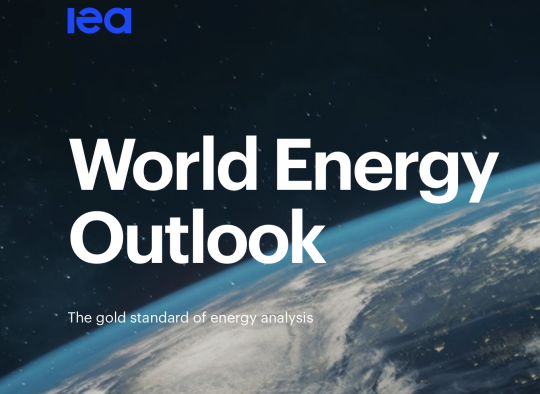
A couple of weeks ago, the International Energy Agency (IEA) released emissions scenarios related to the World Energy Outlook (WEO). We’ve used our spreadsheets to disentangle the drivers for those 2019 scenarios. We express the results in what we call a “dashboard of key drivers” (see below).
The 2019 scenarios are based on IEA’s WEO model, which comes out every year. A related set of scenarios comes from IEA’s Energy Technology Perspectives model. You can read about both sets of analyses here.
For more background, see the post on IEA historical drivers of energy sector carbon dioxide emissions here. For our analysis of key drivers for the 2017 ETP scenario called “Beyond 2 Degrees”, go here.
The reference for our 2019 article [1] upon which the decomposition analysis is based is at the end of this post. Email me for a PDF copy if you can’t get access otherwise. We also have an excel workbook all set up to do these decompositions and graphs, so let me know if you’d like a copy.
Let’s first look at an equation known as the Kaya Identity, which describes fossil carbon emissions as the product of four terms: Population, GDP/person (wealth), Primary Energy/GDP, and Carbon dioxide emissions/primary energy.

Over time, analysts have realized that this four-factor identity collapses some important information. That’s why, in our 2019 article, we moved to the expanded Kaya identity, with several more terms:

The components of this identity are as follows:
CFossil Fuels represents carbon dioxide (CO2) emissions from fossil fuels combusted in the energy sector,
P is population,
GWP is real gross world product (measured consistently using Purchasing Power Parity here and adjusted for inflation),
FE is final energy,
PE is total primary energy, calculated using the direct equivalent (DEq) method (electricity from non-combustion resources is measured in primary energy terms as the heat value of the electricity to first approximation),
PEFF is primary energy associated with fossil fuels,
TFC is total fossil CO2 emitted by the primary energy resource mix,
NFC is net fossil CO2 emitted to the atmosphere after accounting for fossil sequestration.
For historical data, there is no sequestration of carbon dioxide emissions, so the last term is dropped in the historical blog post, but included for future scenarios.
Note that this identity applies only to carbon dioxide emissions from the energy sector. We use an additional additive dashboard for future scenarios to describe industrial process emissions, land use changes, and effects of other greenhouse gases, but IEA doesn’t report all those data, so I’m focusing just on the energy sector here.
Discussion of Figure 1 (Factors)
The first graph is what we call our graph of key factors, from the indented list above. In the first row we show each term in its raw form for both the reference case (in black) and the intervention case (in red). The second row shows indices with 2025 = 1.0. And the last term shows the annual rate of change in each term for reference and intervention cases. In each case, we plot historical trends from IIASA’s PFU database for each factor from 1900 to 2014 (in green dashed lines) and 1995 to 2014 (in blue dashed lines)
The total fossil carbon is the end result of the other factors, which drive emissions. It grows modestly from 2025 to 2040 (when the new IEA scenarios end). This was unexpected for me (similarly to the 2017 ETS scenario), and it suggests an area of fruitful inquiry (and comparison to other reference cases). I would have expected higher growth in emissions in a reference case, but it does indicate some minor (but insufficient) progress in reducing projected emissions growth.
Population doesn’t vary at all between reference and intervention scenarios, which is commonplace for such projections. Population is not seen as a lever for climate policy except in rare cases, mainly for ethical reasons. There may be policies (like educating and empowering women) that we should do for other reasons, but almost never are these considered as climate policies (and that’s appropriate, in my view).
Another observation about population emerges from these data also. Projected population growth to 2040 is slower than historical trends. This result mainly comes from long term changes that almost all demographers agree are underway, and this picture of slowing population growth is almost universal in long run energy scenarios. Unlike the 1971 to 2016 period, when population was responsible for half of growth in energy sector GHGs, this driver will be far less important to emissions in the future.
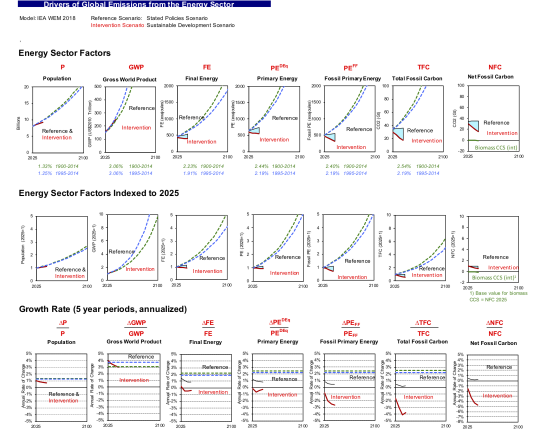
Download higher resolution version of Energy Sector Factors for SDS
Gross World Product (GWP) is another key driver, and that term is projected to increase by more almost three quarters by 2040 in both the reference and intervention cases.
Final energy (e.g., energy consumption measured at the building meter or the customer’s gas tank) is projected to grow modestly in the reference case and decline modestly in the intervention case. Same for primary energy. Both grow much more slowly than historical trends, which is another interesting area of investigation.
Fossil primary energy is roughly constant in the reference case and declines substantially in the intervention case. Same for total fossil carbon and net fossil carbon. Note the green line in the last column for the top two rows, where we plot the contribution of biomass CCS to net emissions reductions in the intervention case for comparison. Though these net emissions savings are often counted outside of the energy sector, they are linked to the energy sector and it’s useful to show their magnitude here for comparison.
The last row of the dashboard shows annual rates of change, which reveal some interesting trends and suggests further investigations. Population grows at a modest but declining rate after 2030. Real GWP growth slows to 2040 but still remains above 3%/year by 2040.
Final energy in the reference case shows modest but declining annual growth rates, while the Intervention case slightly declines over the analysis period.
Primary energy growth rates decline for the reference case and increase for the intervention case (but only after 2030). This means that there are more conversion losses in the energy system over time after 2030 in the intervention case (because final energy growth rates are mostly negative during the forecast period for the intervention case). The effects of these different trends are modest, however.
Fossil primary energy growth is modest over the reference case, but is negative (from -1% to almost -3%/year) for the analysis period. Total fossil carbon and Net Fossil Carbon show modest growth in the reference case and strong annual declines in the intervention case.
Discussion of Figure 2 (Ratios)
The 2nd graph below shows the expanded Kaya identity ratios. Population is the same, but all the other columns show ratios from the 2nd equation above. Population and wealth per person (the first two terms in the Kaya identity) are the biggest drivers of emissions in the reference case, while the energy intensity of economic activity declines to offset some of the growth in the first two terms.
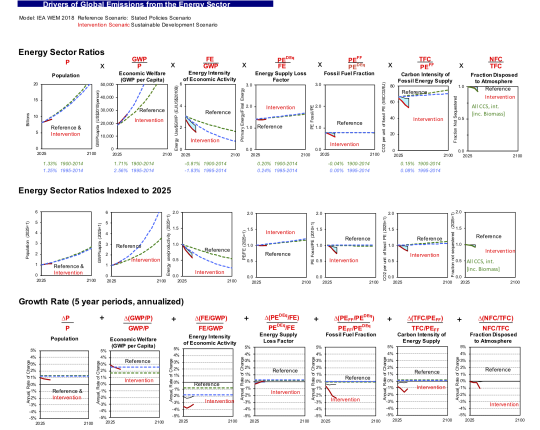
Download higher resolution version of Energy Sector Ratios for SDS
The ratio of final energy to GWP tracks trends since 1995 for the reference case, and declines more rapidly in the intervention case. Why the rate of decline should become more strongly negative to 2030 and then rise again (leading to V-shaped curves for rates of change) is a question worth asking the modelers.
As expected from the discussion above, the energy supply loss factor suggests losses are roughly constant in the reference case and grow by a tiny amount in the intervention case. The fossil fuel fraction (which shows switching from fossil fuels to alternatives) declines substantially over the analysis period, as does the carbon intensity of fossil energy supply (which shows switching among fossil fuels). Interestingly, there’s a step change in the rates of change for the carbon intensity of energy supply in the last five years of the forecast, and it would be interesting to know from the modelers why this comes about.
The last column shows the extent of carbon sequestration as well as carbon sequestration from biomass, which have a modest effect in the Intervention scenario. This column is measured as a fraction of total fossil carbon emitted, so some of the drop in this ratio is associated with declining absolute amounts of fossil carbon over time. Nevertheless, this graph indicates measurable but modest use of carbon sequestration (both conventional and biomass related) in this scenario.
Figure 3: Carbon sequestration in the reference and SDS scenarios
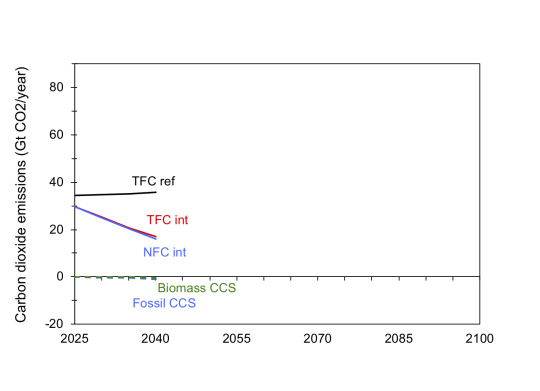
Download higher resolution version of CCS graph
The effect of CCS in the SDS scenario is modest. The difference between TFC reference and TFC Intervention is the result of all factors other than sequestration, and then the tiny difference between TFC Intervention and NFC Intervention is the effect of CCS. We also put absolute reductions from Biomass and Fossil CCS below the zero line. The Biomass CCS effect is close to zero and Fossil CCS effect is small.
Note that IEA does not release CCS results for the Intervention case for 2025 and 2035, only for 2030 and 2040. My colleague Zachary Schmidt fit an exponential curve to the 2030 and 2040 results and used the fitted curve to estimate CCS effects in 2025 and 2035 in the Intervention case.
This example illustrates the use of our decomposition dashboards for the 2019 2019 WEO results. It’s clear from this review that IEA needs to extend its WEO scenario past 2040, which I assume is in the works. It would also be interesting to compare trends in the final energy intensity of economic activity and the fossil fuel fraction to other studies to see whether the IEA projections could become more aggressive.
References
1. Koomey, Jonathan, Zachary Schmidt, Holmes Hummel, and John Weyant. 2019. “Inside the Black Box: Understanding Key Drivers of Global Emission Scenarios.” Environmental Modeling and Software. vol. 111, no. 1. January. pp. 268-281. [https://www.sciencedirect.com/science/article/pii/S1364815218300793]